Mapping Ecosystems with Satellite Data
In 2024, the CEOS Chair, the Canadian Space Agency (CSA), has chosen to prioritise biodiversity monitoring from space. Over the course of 2024, a series of articles will be published here to support the Chair’s goals, and highlight the importance of Earth observations (EO) for Biodiversity. Please see here for other articles in the series.
Space-based observations of land cover and use have served as a proxy for ecosystem mapping for decades, which tend to provide a coarse description of ecosystems that can be insufficient for their management and for meeting Global Biodiversity Framework (GBF) targets.
Ecosystem delineations were traditionally generated through observations of differences in spectral features in land imagery. The increased temporal resolution, availability, and variety of current satellite data have made it possible to measure additional ecosystem characteristics, such as seasonal change patterns, structure, function, and composition. The most common current approach to generating ecosystem maps is based on supervised machine learning, with an emergence in artificial intelligence deep learning processes (for example, see de Sousa et al. (2020)).
In order to measure map ecosystems in-depth, data directly linked to their ecological characteristics is required. These characteristics are grouped into three basic categories of ecosystem properties:
-
- Structure: the spatial arrangement and type of ecosystem components such as canopy height, leaf area index, woody biomass, or canopy layer count.
- Function: the type of ecological process such as productivity, biomass accumulation, or fire dynamics.
- Composition: the species that reside in and form ecosystems, alongside the diversity between them and abundance.
The specific typologies used for ecosystem classification will depend on the region’s ecosystems and desired applications, and will result in varying emphasis among measured ecosystem properties. The International Union for Conservation of Nature (IUCN) Global Ecosystem Typology defines realms, biomes, and ecosystem functional groups, which are used to classify ecosystems based on their functional characteristics. A realm represents one of five primary components of Earth’s biosphere – those applicable to ecosystems are terrestrial, marine, freshwater, and subterranean. Biomes are a subdivision of a realm that describes broad ecosystem structure and major ecological drivers, and ecosystem functional groups are those related within a biome that share ecological drivers. Lower levels classify regional, global, and subglobal ecosystem types.
Earth-observing satellites can be equipped with a variety of sensors designed to differentiate and map Earth surface features. A synergy of sensor technologies and in situ data offer the most comprehensive description of ecosystems and their extent, and are vital for the realisation of EO data for biodiversity and ecosystem applications. Such sensors can be broadly classified into four categories:
- Multispectral
- Hyperspectral
- Synthetic Aperture Radar (SAR)
- Lidar (light detection and ranging)
Multispectral and hyperspectral instruments measure reflected light typically within the visible and near infrared bands (VNIR), as well as the short wave infrared (SWIR) and thermal infrared (TIR) bands. Multispectral sensors capture light in a small number of broad spectral bands (on the order of tens), whereas hyperspectral sensors do so with a large number of narrow and contiguous bands (on the order of hundreds), offering a much finer spectral resolution. Both types of sensor are however limited to viewing only the canopy surface under no cloud cover during day time.
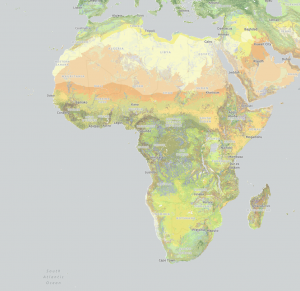
USGS World Terrestrial Ecosystems Explorer captures ecosystems across Africa ranging from Saharan grasslands to Congolian rainforests and South African mangroves. The global dataset houses 431 ecosystem types including both natural ecosystems and converted landscapes.
Multispectral sensors benefit from more extensive heritage and platform support paired with high spatial and temporal resolutions. They provide a basis for characteristics such as vegetation phenology, horizontal structure, and ecosystem productivity dynamics, with global coverage on a near-daily basis.
Hyperspectral data can provide insight into plant functional traits such as canopy physiology and biochemistry, leaf water and chlorophyll content, leaf mass per unit area, and nutrient content. These data can also describe water and atmospheric characteristics, which is derivative of ecosystem health.
SAR sensors are active sensors, which emit microwave radiation toward a source and use the reflected radiation to build topographic imagery. This longer wavelength radiation is unimpeded by clouds, enabling vegetation and terrain structural analyses through cloud cover and at night. The SAR signal penetration depth is dependent on its wavelength. L-band sensors are suited for forest height and biomass mapping. Shorter wavelength C-band sensors can measure surface details at higher resolution at the cost of a lower penetration depth. The shortest X-band SAR sensors are most sensitive to surface topography so find applications in wetland mapping. However, SAR data is quite complex, and hence has proven initially challenging for ecosystem experts to utilise.
Lidar is an active technology like SAR that reflects laser pulses from the ground to derive structural forest measurements such as canopy height and profile. However, it is limited to point-based sampling and has a low heritage across space platforms. The Global Ecosystem Dynamics Investigation (GEDI) mission is a lidar instrument onboard the International Space Station (ISS) that has provided invaluable data for 3D ecosystem structure mapping, demonstrating a need for space-based lidar and global periodic data.
By using all sensor types together with in situ data, a wide array of ecosystem characteristics can be detected, sufficient to distinguish specific types of ecosystems.
More details on mapping ecosystems from space can be explored in the CEOS Ecosystem Extent Task Team’s White Paper ‘Space-based Earth Observation and Ecosystem Extent: Exploring Opportunities’.